Unveiling the Power of Agriculture Datasets for Machine Learning
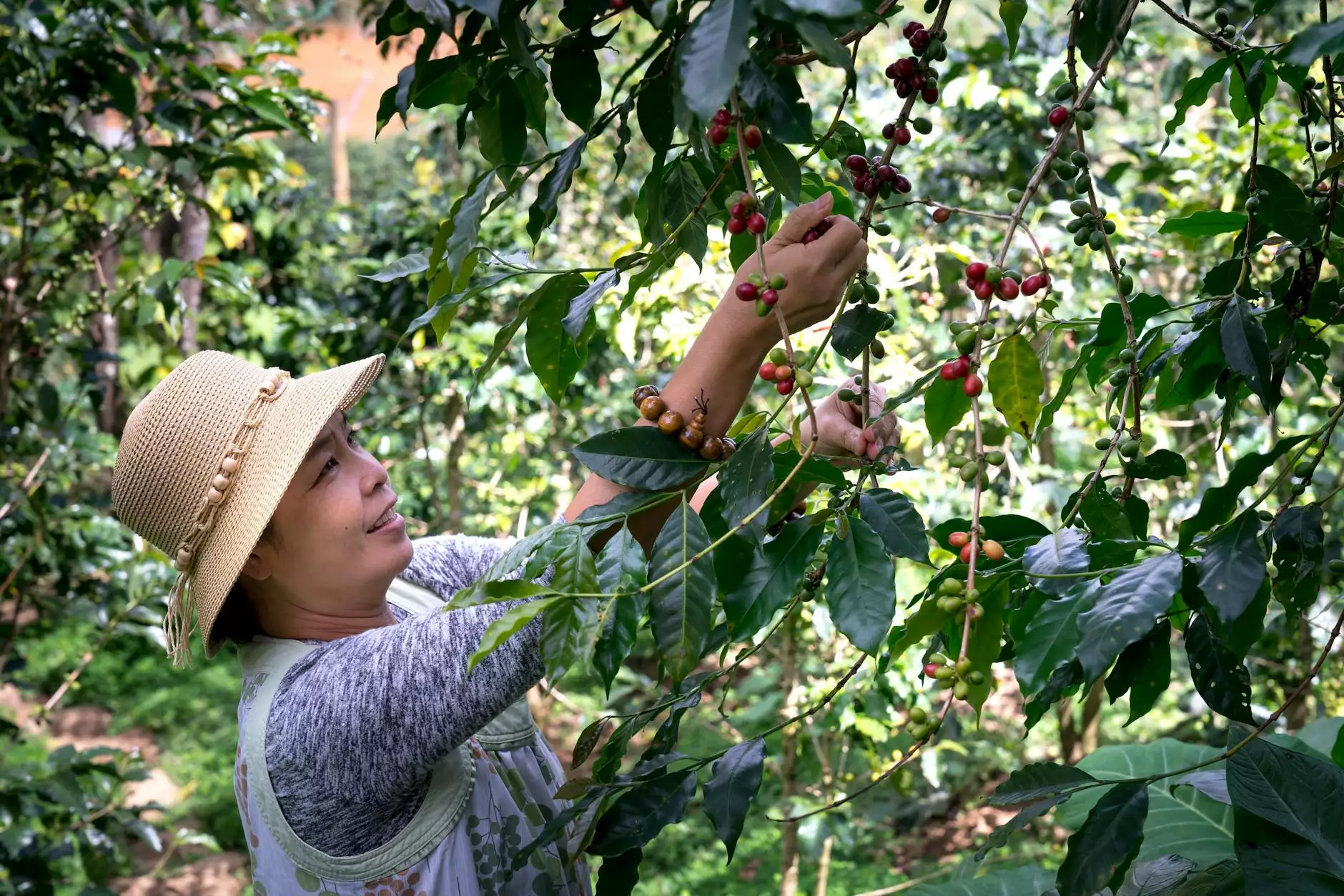
In recent years, the realm of agriculture has undergone a significant transformation thanks to technology and data analytics. Among these advancements, machine learning stands out as a pivotal tool that can help in optimizing farming practices, increasing yields, and managing resources more efficiently. Central to this revolution are agriculture datasets for machine learning, which provide invaluable insights essential for evolving agricultural methodologies.
Understanding Agriculture Datasets
At its core, an agriculture dataset encompasses a collection of data relevant to agricultural practices. These datasets can include various types of information, such as:
- Soil health data: Information about soil composition, nutrient levels, and pH.
- Crop yield statistics: Historical data on crop growth, yield outputs, and quality.
- Weather patterns: Data on temperature, precipitation, humidity, and extreme weather events.
- Pest and disease occurrences: Incidence of invasive pests and plant diseases over time.
- Geographic data: Land use maps and geospatial information for precise farming.
By leveraging these datasets, machine learning algorithms can uncover patterns and make predictive analyses that can streamline farming operations and boost productivity.
Why Are Agriculture Datasets Crucial for Machine Learning?
The significance of agriculture datasets for machine learning comes from their ability to enhance decision-making processes in the agricultural sector. Below are some compelling reasons why these datasets are indispensable:
1. Enhanced Decision-Making
Machine learning models trained on agriculture datasets can provide actionable insights that enable farmers to make informed decisions regarding planting, irrigation, pest control, and harvesting. Data-driven decisions lead to more optimized operations.
2. Predictive Analytics
Using historical data, machine learning models can forecast future events such as crop yields, pest invasions, and optimal planting times. These predictions help farmers allocate resources effectively and mitigate risks associated with extreme weather or pest outbreaks.
3. Resource Optimization
Farmers can significantly reduce wastage of water and fertilizers by using machine learning applications powered by agricultural datasets. These technologies allow for precision farming, which means that resources are utilized only where and when needed.
4. Sustainability
With the growing concern for sustainable practices, machine learning in agriculture, fueled by rich datasets, can help in creating sustainable farming methods that reduce the environmental impact while maximizing output.
Types of Agriculture Datasets Available
The variety of datasets available for machine learning applications in agriculture is vast. Here are some major types:
1. Remote Sensing Data
Remote sensing involves collecting data via satellites or drones. This data helps in assessing crop health, moisture levels, and land usage. Remote sensing data is vital for precision agriculture and the development of models that predict crop conditions.
2. Experimental Research Data
Field experiments conducted by agricultural researchers produce datasets that incorporate variables like crop varieties, soil treatments, and climate conditions. These datasets help in understanding the impacts of different farming techniques on crop yield.
3. Open Datasets
There are numerous publicly available datasets provided by various organizations and research institutions. These datasets can serve as a starting point for machine learning applications and can be found at repositories like Kaggle and data.gov.
4. Historical Yield Data
Collected over several years, this data includes historical performance information of crops. It is essential for training predictive models related to yields under different climatic scenarios and management practices.
Challenges in Utilizing Agriculture Datasets for Machine Learning
While there's immense potential in utilizing agriculture datasets for machine learning, it's not without its challenges:
1. Data Quality
The accuracy and reliability of datasets can vary significantly. Inconsistent data can lead to erroneous conclusions and ineffective predictions. It's crucial to ensure that the data is robust and representative of real-world conditions.
2. Data Integration
Data comes from various sources and in different formats, making integration a challenging process. To build effective models, datasets must be harmonized and consolidated into a usable format.
3. Interpretability of Models
Machine learning models, especially deep learning algorithms, can act as "black boxes" where decision-making processes aren't easily interpretable. This lack of transparency can be an obstacle in gaining farmer trust and adoption.
4. Scalability
Models that work well on small datasets may not perform similarly on larger scales. Ensuring that machine learning applications can scale to various farm sizes and types is essential for broader adoption.
Best Practices for Implementing Machine Learning in Agriculture
To maximize the benefits of agriculture datasets for machine learning, consider these best practices:
1. Start with a Clear Objective
Clearly define the problem you are trying to solve with machine learning. Whether it's optimizing irrigation schedules or predicting pest outbreaks, having a clear objective will guide your dataset selection and model choice.
2. Utilize Domain Knowledge
Incorporating insights from agronomists and agricultural scientists can greatly enhance the quality and relevance of the machine learning model. Their expertise will help identify the most critical variables to include in the analysis.
3. Ensure Data Quality
Before feeding data into your models, it's essential to clean and preprocess it. This includes removing outliers, filling in missing values, and standardizing the dataset to ensure high-quality outputs.
4. Continuous Learning and Adaptation
Machine learning models should be continuously refined as new data becomes available. Regularly updating the models in response to feedback and new agricultural practices is key to maintaining accuracy.
The Future of Agriculture Datasets and Machine Learning
The trajectory of machine learning in agriculture is on an upward trend, thanks to the constant development and availability of agriculture datasets for machine learning. As technologies advance, we can expect:
1. Increased Automation
Farmers may implement more automated systems powered by robust data analytics and machine learning algorithms, allowing for hands-free interventions and monitoring of crop health.
2. Personalized Farming Solutions
With machine learning, solutions can be tailored to individual farms based on specific datasets, improving the effectiveness of recommendations and interventions.
3. Collaborative Data Platforms
The emergence of platforms that facilitate data sharing among farmers can lead to richer datasets, providing a more comprehensive understanding of agricultural trends and practices.
4. Enhanced Climate Resilience
As climate change poses a significant threat to global agriculture, machine learning models powered by quality datasets will play a crucial role in helping farmers adapt to changing conditions and mitigate risks.
Conclusion
In conclusion, the integration of agriculture datasets for machine learning presents an exciting frontier for the agricultural industry. By harnessing the power of data and machine learning, farmers can optimize their practices, improve yields, and contribute to sustainable food production. As technology continues to evolve, embracing these innovations will be essential for ushering in a new era of agriculture that prioritizes efficiency, sustainability, and resilience.
Get Started with Keymakr.com
If you're looking to dive deeper into the world of machine learning and agriculture datasets, Keymakr.com offers advanced software development solutions tailored for the agricultural sector. Our expertise can help you leverage data to transform your agricultural practices effectively. Contact us today to learn more!
agriculture dataset for machine learning