The Future of Data Annotation: Labelling Tool Machine Learning
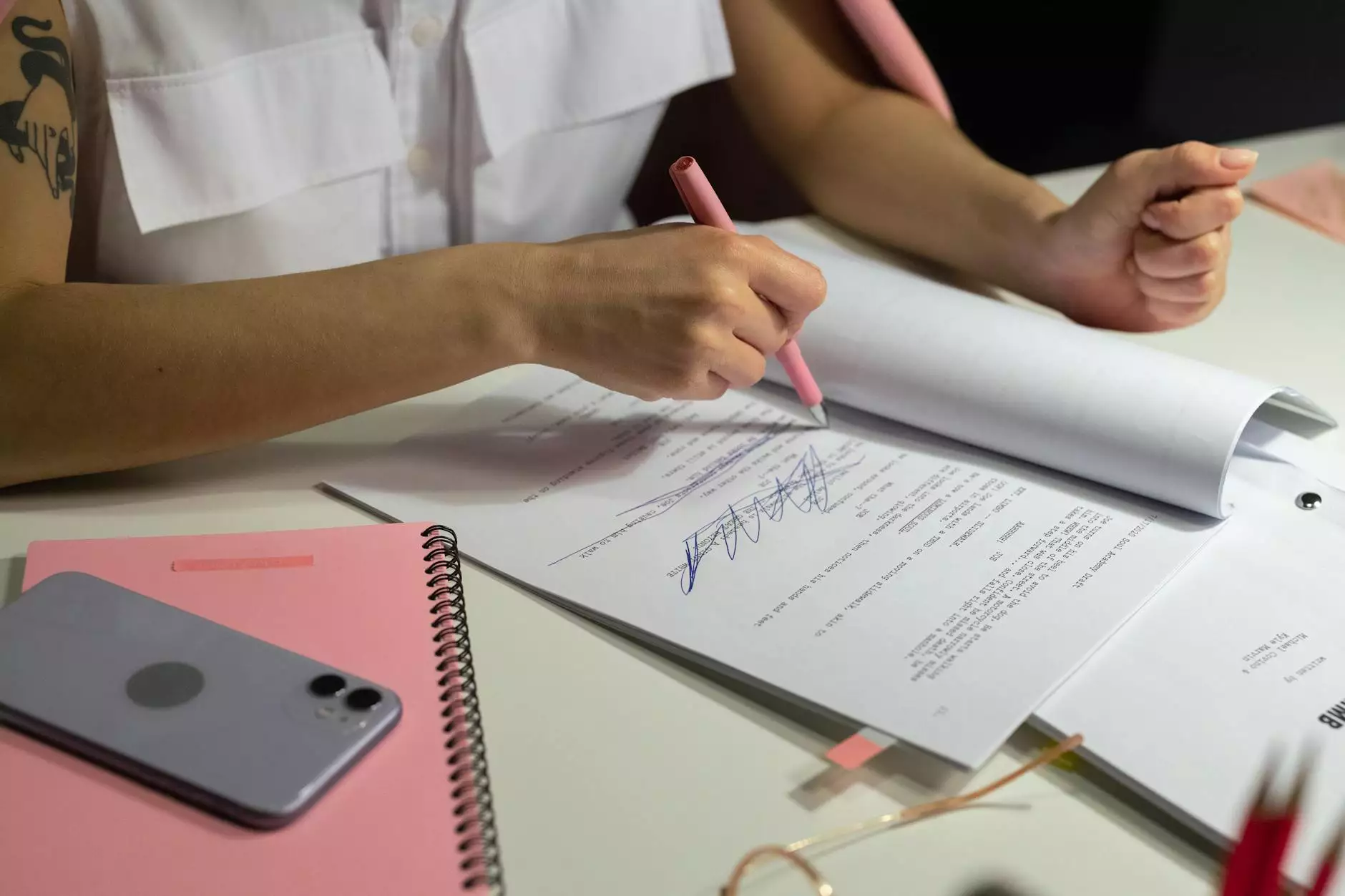
In the modern landscape of artificial intelligence (AI) and machine learning (ML), data quality is paramount. As we venture into an era dominated by big data, the necessity for effective data annotation has never been more significant. One of the most crucial elements in this process is the labelling tool machine learning. In this article, we will explore the intricate workings of labelling tools, their impact on data annotation platforms, and how businesses can leverage them to enhance operational efficiency.
Why Labelling Matters in Machine Learning
Labelling is essentially the process of assigning meaningful tags or classes to data. This phase is vital for training machine learning models, as it provides the foundational information that enables algorithms to learn from data. The accuracy of these labels directly influences the end performance of a machine learning model.
- Quality Control: Consistently high-quality labels contribute to robust and reliable machine learning outputs.
- Model Accuracy: Accurate labelling increases the precision and recall rates of models.
- Adaptability: Well-labeled data enhances a model's ability to generalize to new, unseen data.
Understanding Labelling Tool Machine Learning
The labelling tool machine learning integrates sophisticated algorithms to aid in the annotation process. These tools are designed to automate and streamline data labelling, significantly reducing the time and effort required from human annotators. By leveraging various techniques, these tools aim to provide accurate, consistent, and customizable data labels.
The core functions of these tools can be summarized as follows:
- Automated Annotation: Using machine learning, automated systems can predict the labels for new data based on previous examples.
- Human Review: Despite automation, human oversight remains critical for ensuring label quality, allowing for corrections and adjustments as necessary.
- Scalability: ML-based labelling tools can handle vast amounts of data, accommodating businesses of all sizes, from startups to enterprises.
Types of Labelling Tools in Machine Learning
There are various types of labelling tools employed in machine learning, each designed to cater to specific data types and application domains:
1. Text Annotation Tools
Used primarily for natural language processing (NLP) applications, these tools assist in labelling text data with sentiment, named entities, and more.
2. Image Annotation Tools
These tools are pivotal in computer vision projects, enabling users to annotate images with bounding boxes, segmentation masks, and key points.
3. Audio Annotation Tools
For applications involving audio data, such as speech recognition, these tools label audio segments for transcription, sentiment analysis, etc.
4. Video Annotation Tools
Used for labeling video frames, these tools help in identifying objects, actions, or events within video data.
Utilizing Labelling Tool Machine Learning in Your Business
As organizations delve into data-driven decision-making, the integration of labelling tool machine learning can revolutionize the way businesses handle their data annotation tasks.
Improved Efficiency
By automating a significant portion of the labelling process, businesses can free up human resources for more complex tasks, leading to enhanced workflow efficiency.
Cost-Effectiveness
Investing in automated labelling tools can significantly reduce costs associated with manual annotation, particularly for large datasets requiring constant updates.
Enhanced Accuracy
Implementing machine learning-based labelling tools helps ensure that data is labelled consistently, which can lead to improved model performance and overall project success.
Challenges and Considerations
While the benefits of labelling tool machine learning are substantial, there are challenges that businesses must consider:
- Initial Setup Costs: There may be upfront costs associated with implementing sophisticated labelling tools.
- Training Requirements: Teams may require training to effectively use and adjust the tools to fit their specific needs.
- Quality Assurance: Even with automation, regular checks are necessary to maintain annotation quality.
The Future of Data Annotation with Machine Learning
As technology evolves, we can anticipate significant advancements in labelling tools through machine learning. Several trends are emerging:
1. Increased Integration with AI
Future labelling tools will leverage deeper integration with artificial intelligence, allowing for more nuanced automated labelling processes.
2. Enhanced Collaboration Features
Tools are beginning to utilize collaborative frameworks that allow multiple users to annotate data simultaneously, improving speed and efficiency.
3. Customizable Machine Learning Models
Businesses will have the ability to adapt and customize machine learning models within these labelling tools based on specific project requirements, enhancing relevance and accuracy.
Conclusion
As companies continue to harness the power of data, the significance of high-quality labelling becomes increasingly clear. The labelling tool machine learning stands at the forefront of this evolution, providing businesses with the capability to streamline processes, improve data accuracy, and ultimately drive better business outcomes. By adopting these innovative tools, organizations can not only enhance their machine learning models but also empower their data annotation efforts on platforms like KeyLabs.ai.
The time to embrace the future of data annotation is now with labelling tool machine learning paving the way for innovation, efficiency, and accuracy in the world of AI and beyond.