Maximizing Potential with Annotated Image Datasets
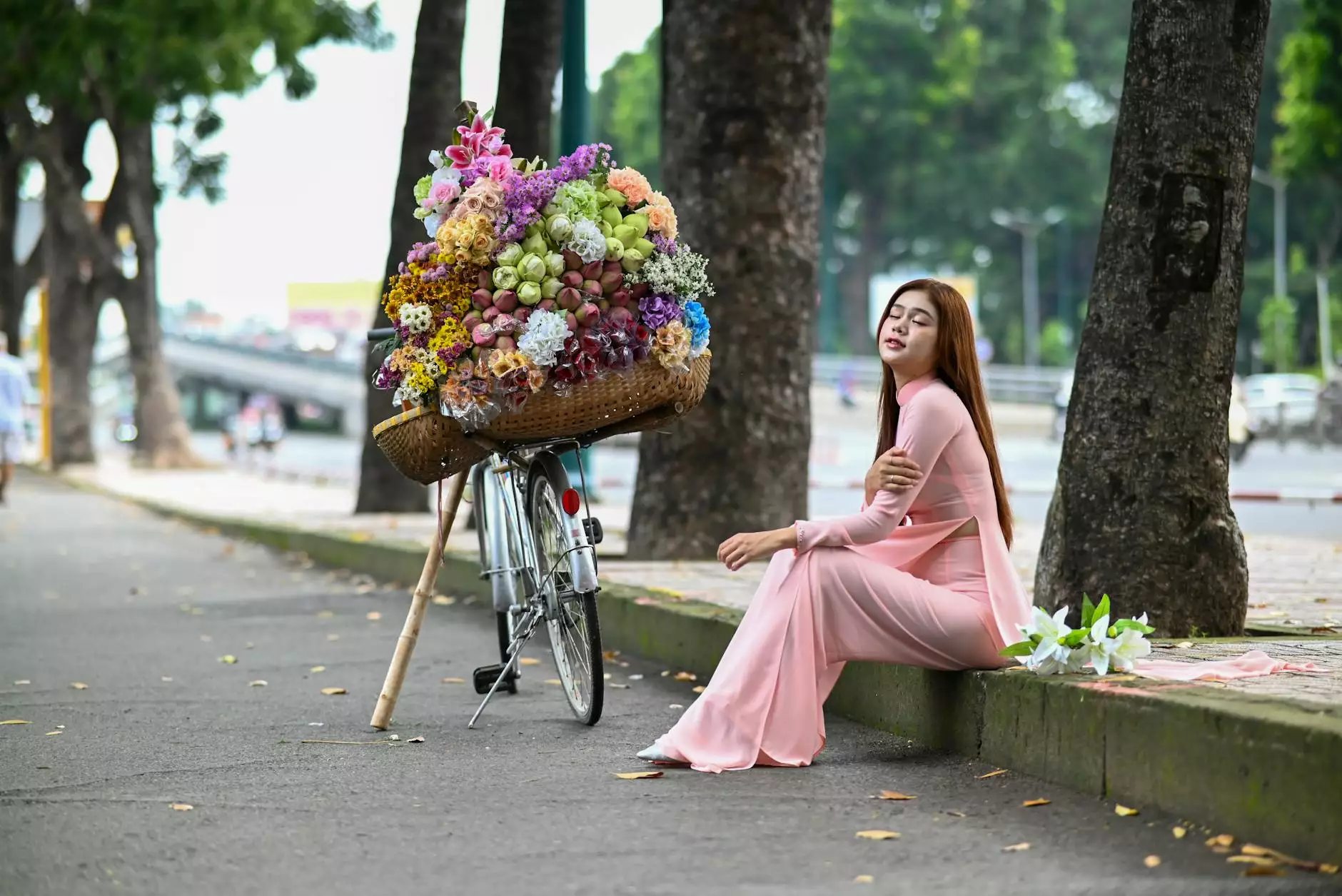
The emergence of artificial intelligence (AI) and machine learning (ML) has revolutionized various industries, leading to a significant demand for high-quality, annotated image datasets. Annotated image datasets are crucial for training models that can accurately interpret and respond to visual data. In this article, we will delve into the importance of annotated image datasets, the tools and platforms available for data annotation, and how businesses can optimize their operations through these valuable resources.
Understanding Annotated Image Datasets
At its core, an annotated image dataset is a collection of images that have been tagged or marked up to indicate specific features, objects, or attributes within the images. This process of annotation helps machine learning algorithms learn from the data, allowing them to make accurate predictions or classifications in real-world applications.
Why Annotation is Essential
The primary objective of data annotation is to produce a dataset that can teach AI and ML algorithms to recognize patterns and make informed decisions. Without properly labeled data, even the most advanced neural networks will struggle to perform effectively. The significance of high-quality, annotated image datasets cannot be overstated as they serve as the foundation for various applications, including but not limited to:
- Autonomous Vehicles: Critical for object detection and navigation.
- Healthcare: Aiding in diagnostics through image analysis.
- Retail: Enhancing image recognition for inventory management.
- Security: Developing surveillance systems that can accurately identify intruders.
Types of Data Annotations
Data annotation encompasses various methods, each tailored to specific needs and use cases. The common types of annotations for images include:
1. Bounding Boxes
This method involves drawing rectangles around objects of interest in an image. It's widely used in tasks such as object detection, where the goal is to locate and recognize multiple objects within a single image.
2. Semantic Segmentation
In semantic segmentation, each pixel is classified into a category, allowing for finer detail in recognizing objects. This is particularly useful in applications like scene understanding and autonomous driving.
3. Key Point Annotation
Key point annotation marks specific points or features in an image, often used in human pose estimation or facial recognition tasks.
4. Polygonal Segmentation
Polygonal segmentation allows for more complex shapes than bounding boxes, providing more accurate representations of objects with irregular outlines.
Why Choose Keylabs.ai for Data Annotation?
When it comes to sourcing annotated image datasets, businesses face the challenge of selecting an efficient, reliable, and high-quality data annotation service. Keylabs.ai stands out in the crowded marketplace with its innovative approach to data annotation, offering many benefits:
1. Advanced Technology
Keylabs.ai employs state-of-the-art tools that enhance the efficiency and accuracy of the annotation process. Our data annotation tools are designed to handle large volumes of data with precision.
2. Expert Team
Our team of highly skilled annotators is trained to ensure that every image is annotated to the highest standards. This expertise significantly reduces the chances of errors in datasets.
3. Scalability
As businesses grow, so do their data needs. Keylabs.ai offers scalable solutions that can accommodate projects of any size, from small datasets to extensive image libraries.
4. Rapid Turnaround Time
With streamlined processes and advanced technology, we provide a rapid turnaround time for annotated image datasets, helping you keep your projects on schedule.
Creating Your Own Annotated Image Dataset
While partnering with a service like Keylabs.ai provides many advantages, some businesses may prefer to create their own annotated image dataset. Here are the steps to follow:
Step 1: Define Your Objectives
Determine the specific tasks your model will perform. The kind of annotation you choose will depend on your objectives, whether it be object detection, image classification, or something else.
Step 2: Collect Raw Images
Gather the images you need for your dataset. Ensure you have a diverse range of images that represent various scenarios your model will encounter.
Step 3: Choose an Annotation Tool
Select a reliable tool for annotating the images. Keylabs.ai offers several tools that you can utilize for this purpose, designed to facilitate various types of annotations.
Step 4: Start Annotating
Begin the annotation process by marking objects, shapes, points, or areas based on your chosen method. It’s vital to maintain consistency in how annotations are applied to ensure reliable model training.
Step 5: Quality Assurance
After annotating your images, conduct thorough quality checks to rectify any inconsistencies or errors before using the data for training.
Best Practices for Annotating Image Datasets
Effective annotation is essential for the success of machine learning models. Here are some best practices to keep in mind:
- Clear Guidelines: Create comprehensive guidelines for annotators to ensure consistency across the dataset.
- Regular Review: Implement a review process where annotations are consistently checked for accuracy.
- Use Tools Wisely: Leverage automation features in annotation tools to improve efficiency while ensuring high-quality outputs.
- Maintain Diversity: Ensure your dataset includes a variety of images to enhance the model’s ability to generalize in real-world scenarios.
- Document Everything: Keep thorough documentation of the dataset, annotations, and any changes made during the process.
The Future of Annotated Image Datasets
The future of annotated image datasets looks promising, driven by advancements in technology and an increasing reliance on AI in everyday life. As machine learning models become more sophisticated, the demand for high-quality annotated datasets will only rise. Businesses that invest in acquiring and utilizing well-annotated datasets will position themselves as leaders in innovation and efficiency.
Emerging Trends in Data Annotation
Several trends are shaping the future landscape of how we create, utilize, and understand annotated image datasets:
- Automation: Machine learning and AI are being leveraged to automate parts of the annotation process, significantly speeding up data preparation.
- Crowdsourcing: Platforms that utilize crowdsourcing for data annotation are becoming more popular, allowing organizations to tap into a wider workforce.
- Real-time Annotation: Innovations in real-time annotation tools are allowing for faster feedback loops and immediate data usage.
- Quality Control Through AI: Using AI to detect annotation errors and improve quality control is becoming standard practice.
- Enhanced Collaboration: Cloud-based platforms enable better collaboration between teams working on data annotation, improving efficiency and output quality.
Conclusion
In conclusion, annotated image datasets are an integral part of the AI and machine learning landscape. Businesses can maximize their potential by understanding the importance of high-quality data annotation, the tools available, and best practices involved in the process. With services like Keylabs.ai, companies can ensure they harness the power of AI effectively, leading to knowledgeable decisions and innovations that better serve their clients and market needs.
To stay ahead in the competitive market, invest in high-quality annotated image datasets today, and see how they transform your business capabilities!